Hamed Ebrahimian
Ph.D., P.E.
Assistant Professor
Department of Civil and Environmental Engineering
University of Nevada, Reno (UNR)
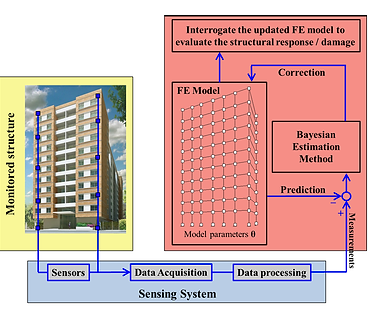

Structural Health Monitoring and Damage Identification of Civil Structures Based on Nonlinear Finite Element Model Updating using Bayesian Inference Framework
Through this research, which was one of the innovations of my Ph.D. work, we proposed, developed, and initially validated a new structural health monitoring (SHM) methodology for post-disaster safety monitoring and damage identification (DID) of civil structures. This innovative methodology consists of stochastic updating of a nonlinear mechanics-based finite element (FE) model of the structure using the dynamic input-output data measured during a damage-inducing event. Recognizing damage as various manifestations of hysteretic material nonlinearity, the updated FE model can be used to reconstruct the response behavior of the structure and therefore, to identify and quantify the state of damage throughout the system.
Two novel frameworks were proposed and developed for nonlinear system and damage identification of civil structures to estimate the FE model parameters, and update the FE model of the structure of interest. The first framework was based on a recursive estimation approach and used the extended and unscented Kalman filters to estimate the FE model parameters. The second framework was based on a batch Bayesian estimation approach and results in an extended maximum likelihood estimation to jointly update the FE model parameters and identify measurement noise amplitude. This research established an initial framework for SHM and DID that can be applied to real-world civil structures. We have published three journal papers on this subject along with a number of conference papers.